Microfluidics for cell culture applications has seen tremendous growth in the last 20 years. In the quest for greater physiological relevance, microfluidic 2D cell cultures gave way to 3D cell cultures which eventually led to organ on chips (OOC’s), also known as microphysiological systems (MPS) or tissue chips1. As the name implies, such systems aim to recapitulate organ-level function by recreating functional units of organ systems using a microfluidic device or platform that enables the following:
- Seeding of organ-specific primary or induced pluripotent stem cell (iPSC) derived cells in a manner that encourages cells to assemble into physiologically relevant configurations that matches the functional units of native organ systems; this can include multiple cell types to account for the role played by different cells present in each organ as well as extra-cellular matrix.
- Perfusion with appropriate media to nourish cells, transport waste, supply/maintain oxygen levels and apply appropriate shear stress; in many systems, this includes a parenchymal compartment and a vascular compartment to mimic vascularization2.
- Providing organ-specific stimuli. e.g.: electrical stimuli for heart on chips, mechanical strain for lung on chips and other organs that require contraction/expansion.
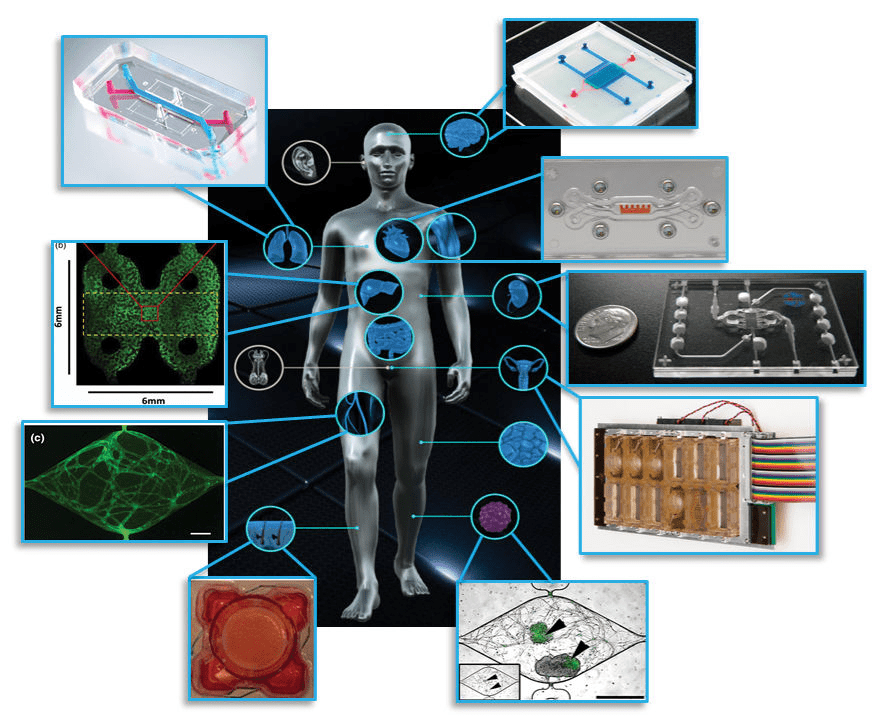
Figure 1: Examples of organ on chip systems that have been developed include (clockwise from top right) a blood-brain barrier (Wikswo lab at Vanderbilt University), cardiac muscle (Parker lab at Harvard), kidney proximal tubule (www.nortisbio.com ), female reproductive tract (DRAPER laboratories), vascularized tumor (George lab at Washington University), skin epidermis (Christiano lab at Columbia), vasculature (George lab at Washington University), liver (Taylor lab at University of Pittsburgh), and lung (www.emulatebio.com). Center image from www.ncats.nih.gov/tissuechip. Figure is taken from1.
Various organ systems have been modelled via OOC’s using these principles (refer Figure 1) and have been covered in-depth elsewhere3. OOC platforms provide greater insight into fundamental organ biology and can model how diseases develop and progress, leading to improved therapeutics and treatment strategies1. The potential applications of OOC’s in basic, translational, and clinical research including regenerative medicine and personalized medicine4 has spurred great interest from researchers and funding agencies5. Meanwhile, the positioning of OOC’s as improved pre-clinical testing platforms that can augment existing animal models and eventually replace them has caught the attention of pharmaceutical companies and regulatory bodies6.
The current gold standard for pre-clinal testing has been animal models, for which there exists many decades worth of data to fall back on. While no one will argue that animal models are ideal, they are still the best method currently available for pre-clinical testing, short of testing on humans. This lack of a better model is what helped drive research into microfluidic 3D cell cultures and OOC’s forward as an alternative to animal testing. The biggest argument for OOC’s over animal models (besides the major ethical debate over breeding animals solely for sacrificing in drug tests) is that they use human cells and model human organ function – factors that should make them a better predictive model than animals. However, since OOC’s are so new and unproven (with the kind of rigour that pharma and regulatory bodies expect), there is a fair amount of healthy skepticism that must be overcome. Some of the big questions being asked by pharmaceutical companies with regards to OOC’s are:
- How translatable and scalable are OOC’s outside of the labs in which they are developed? Proof of concept demonstrations with low number of replicates carried out in one lab can look very promising, but how well can these be deployed in large numbers by different groups of peoples at different labs/institutes while still providing the same quality of data?
- What are the benchmarks used to evaluate OOC’s – against each other and against animal and patient data? The lack of a standardized set of parameters by which OOC’s can be evaluated makes it difficult to compare OOC performance and identify strengths and weaknesses.
- How valid are the predictive capabilities of these systems? How much credibility can be associated with what an OOC says about toxicity or efficacy of a new drug candidate – especially if there is conflicting data from existing preclinical models?
- In what context can OOC models be used – can they replace animal studies or complement them? Or are they better suited for mechanistic studies? How will regulatory bodies accept data coming from OOC models?
The above questions are not trivial, and many ongoing discussions between academics, regulatory bodies, and industry are already taking place to address these issues6,7. The remainder of this article looks only at the first point raised: the transferability and scalability of OOC’s. This might at first glance appear to be a simple logistical problem; however, the complexity of OOC’s turns this into a much more complicated issue.
OOC-based experiments in academic labs are done at a low scale with most labs running 8 – 20 OOC’s in parallel at any given time. Thus, each OOC receives significant attention every step of the way from highly specialized personnel who can take preventive steps at the first sign of trouble if needed. Given the relatively small number of replicates and the proof of concept nature of the work, entire experiments can be repeated if significant issues arise. However, in highly regulated healthcare environments, for results to be accepted as valid, significant confidence must be established in the testing platform’s ability to provide repeatable, reproducible and consistent results with many replicates. Repeating entire batches of experiments or devoting excessive resources to maintain and monitor each individual OOC does not become feasible in such situations. For OOC’s to move into the mainstream as a standardized tool, they will have to become more “plug and play” – easy to set up in large numbers and maintain without requiring troubleshooting at every step of the way. Bottlenecks that can get in the way of scaling up OOC platforms can arise from:
-
The microfluidic device/platform:
Most academic OOC platforms use custom microfluidic devices made in-house using soft lithography and PDMS. While this allows complex devices to be made, batches are small and yield rates low – issues which can be tolerated in proof-of-concept platform demonstration situations where replicates are low but untenable in higher throughput rigorous screening scenarios. Translating PDMS designs to commercially viable plastic or glass devices that can be mass fabricated comes with its own set of challenges (e.g. intricate internal features that can be cast in PDMS are harder to translate to plastics/glass, PDMS is oxygen permeable, while most plastics and glass are not, organ-specific stimuli designed in PDMS might not readily translate to other materials, etc.) Thus, developing a microfluidic device or platform that can be fabricated in large batches with high yield rates, while still enabling the OOC to be established and maintained is usually the first obstacle for scalability. To address these needs, commercial microfluidic platforms targeted at OOC’s are being developed or already available. However, these tend to lock down a particular OOCmethod/approach/design, leaving room for multiple competing platforms to be developed if they can demonstrate competitive advantages (e.g.easier to setup/maintain, lower cost, higher throughput, or improved functionality)
-
Sourcing the cells:
Most OOC’s rely on either primary human cells or iPSC derived cell types. This represents the biggest bottleneck in scaling up OOC’s since primary human cells are scarce and variable, while current iPSC differentiation protocols can provide sufficiently mature cells for only a few organs (e.g.: brain, heart)8. Even with iPSC derived cells considered sufficiently mature for use in OOC’s, differentiation protocols can be lengthy with up to months at a time needed to produce adult-like cells that can still have significant batch-to-batch variability and throw off OOC performance benchmarks. Moreover, there is the natural variation and heterogeneity between cells, organs, and individuals9 that adds significantly to the complexity of developing OOC’s and understanding what exactly represents normal response and what falls into aberrant behaviour. While initial OOC’s started off by limiting themselves to one particular lot of primary cells or one particular source and protocol for iPSC cells to enable comparisons, how will measurements and performance be affected when incorporating cell types from different donors or sources? Thus, cells represent the greatest source of uncertainty when attempting to scale up OOCs10. For large scale deployment, initial solutions will most likely lie in biomanufacturing iPSC’s with well-defined differentiation protocols that have been rigorously tested and validated. Incorporating donor-specific cells for precision medicine applications though, will require further work to ensure differentiation protocols for patient-derived iPSC’s yield cells that match the patients’ own native organ cells, and ensuing OOC performance matches the patient donor organ performance before trying to develop personalized treatment strategies based off OOC predicted results.
-
Reliable protocols for setting up and maintaining OOC’s:
The importance of having well defined and documented protocols while setting up and establishing OOC’s cannot be overstated. Wherever possible, processes should be quantified and/or described with technical parameters to take out any guesswork. Leaving room for interpretation by personnel while setting up OOC’s can be a recipe for disaster, since even slight ambiguities in areas such as the method of seeding cells, injecting ECM or setting up perfusion can have dramatic implications on cell distribution, viability, and function. Protocols must also consider practical issues that may be encountered, such as how to deal with bubbles, collect efflux samples or prepare chips for imaging
-
Thorough validation of the entire system:
This is an obvious requirement since users need to have confidence in the overall test platform and the results it generates; wide variations in OOC responses from device to device or batch to batch can erode trust in the usefulness of the platform. Thus, rigorous evaluations of the entire OOC must be done to benchmark performance, establish normal baseline behaviour, and how to interpret and identify changes to lead to valid conclusions.
While the above points do not capture the entire range of issues with scaling up OOC’s, they do highlight some of the initial steps to be considered. The field of OOC’s is one that is continuing to grow and evolve at a rapid pace. However, it is still important to examine how end-users use and deploy OOC’s in their venues to then draw insights into remaining questions related to ease of use, practicality, and context of use. For this to occur, OOC’s need to be scaled up and translated from academic labs to end-user settings. Ultimately, the OOC that gets adopted might not be the one that is the most advanced, but the one that can be easily set up and replicated while still providing compelling organ-like data.
References
1. Low, L. A. & Tagle, D. A. Tissue chips – innovative tools for drug development and disease modeling. Lab Chip17, 3026–3036 (2017).
2. Osaki, T., Sivathanu, V. & Kamm, R. D. Vascularized microfluidic organ-chips for drug screening, disease models and tissue engineering. Curr. Opin. Biotechnol.52, 116–123 (2018).
3. Ronaldson-Bouchard, K. & Vunjak-Novakovic, G. Organs-on-a-Chip: A Fast Track for Engineered Human Tissues in Drug Development. Cell Stem Cell22, 310–324 (2018).
4. Low, L. A. & Tagle, D. A. ‘You-on-a-chip’ for precision medicine. Expert Rev. Precis. Med. Drug Dev.3, 137–146 (2018).
5. Zhang, B. & Radisic, M. Organ-on-a-chip devices advance to market. Lab Chip17, (2017).
6. Livingston, C. A., Fabre, K. M. & Tagle, D. A. Facilitating the commercialization and use of organ platforms generated by the microphysiological systems (Tissue Chip) program through public-private partnerships. Comput. Struct. Biotechnol. J.14, 207–210 (2016).
7. Willyard, C. Channeling chip power: Tissue chips are being put to the test by industry. Nat. Med.23, 138–140 (2017).
8. Low, L. A. & Tagle, D. A. Microphysiological Systems (Organs-on-Chips) for Drug Efficacy and Toxicity Testing. Clin. Transl. Sci.10, 237–239 (2017).
9. Mertz, D. R., Ahmed, T. & Takayama, S. Engineering cell heterogeneity into organs-on-a-chip. Lab Chip18, 2378–2395 (2018).
10. Sakolish, C. et al. Technology Transfer of the Microphysiological Systems: A Case Study of the Human Proximal Tubule Tissue Chip. Sci. Rep.8, 14882 (2018).
Enjoyed this article? Don’t forget to share.
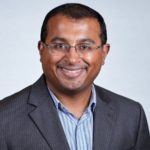
Subin M. George
Subin George has a decade of biomedical research experience spanning digital microfluidics for 3D cell culture, liver microphyiological systems, and implantable sensors for non-invasive continuous monitoring of oxygen, glucose and lactate. In the process, he acquired significant inter-disciplinary experience and enjoys working with research professionals from various technical backgrounds. His current interests lie in translating biomedical research from proof of concept settings to real-world applications. Apart from research, he enjoys spending time with his wife and daughter, catching up with technology, cooking, hiking, and playing soccer.