Personalized cancer therapy is a treatment strategy based on the ability to predict which patients are more likely to respond to specific cancer therapies1. Different factors such as tumor biophysical markers, tumor site, patient genetic factors, and characteristics need to be considered in determining a specific therapy for a patient. Tumor biophysical markers including phenotypes and genotypes are associated with patient prognosis and response to therapy as phenotypes like size, shape, stiffness of individual cells within a tumor can be correlated with the state of the disease and genotypic information from DNA, RNA and protein sequencing can reveal genomic alterations. Moreover, patient genetic factors are also important as they can be associated with drug metabolism, drug toxicity, and overall drug response.
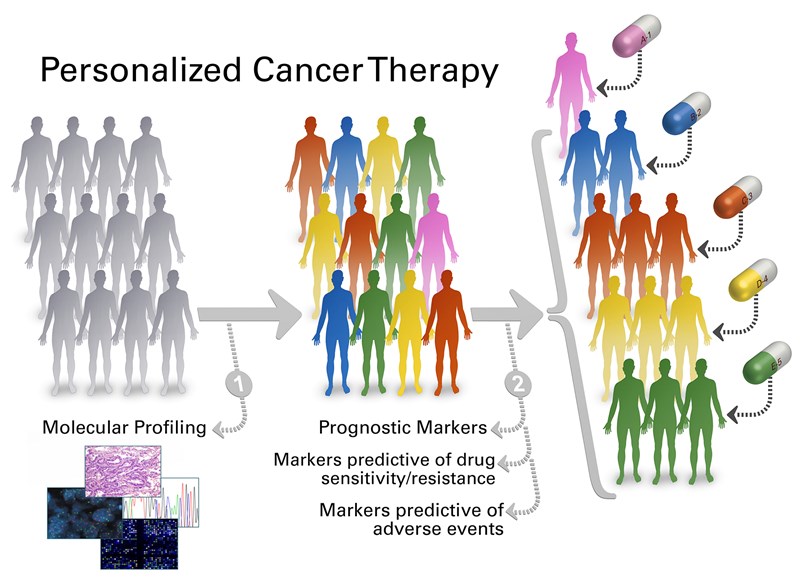
Figure 1. Personalized cancer therapy1. Credit: The University of Texas MD Anderson Cancer Center
The field of microfluidics has come a long way after the introduction2 in the early 1980s and found its application in a wide range of fields like molecular biology, cell biology, genomics, proteomics, astrobiology, optics, and fuel cells. Microfluidics also has shown great promise for cancer diagnosis and in understanding cancer biology with its inherent advantage of high sensitivity, high throughput, less sample consumption, low cost, and enhanced spatio-temporal control3. Microfluidic tools developed over the years for probing cancer have dealt with a small part of the big problem like isolating cancer cells from biopsied tissue sample or patient-derived bio-fluids (blood, pleural effusion), phenotype individual cells in terms of size, shape and deformability, extract the genetic materials like DNA, RNA and proteins from cells and sequencing them, culture cancer cells inside the microfluidic chip, analyze drug dose response etc. Now is the time we should think of combining the pieces together and develop a complete, automated process for personalized cancer therapy.
An outright microfluidic tool for personalized cancer therapy should integrate all available individual tools and automate the process. This tool should have the capability to analyze single cells at a high throughput fashion to tackle the heterogeneity within a tumor. I believe, it will be surely very challenging but not impossible. Isolating cancer cells from patient samples is the first step of the process. The patient sample is either a piece of biopsied tissue or bio-fluids like blood or pleural effusion. The device should have the capability to isolate cancer cells from those samples. Sanjin et al4. has published a nice review article on sample preparation for single-cell analysis in microfluidics. Hattersley et al5. has shown the capability of microfluidics in maintaining a viable tissue sample for 72 hours in the device and then dissociate it to get 78±2.4% viable single cells.
Although the isolation of cancer cells (known as circulating tumor cells, CTCs) from blood is very difficult, mainly because of their insignificant numbers (1-10 CTCs per billions of blood cells in 1 mL of blood), noninvasive, label-free microfluidic tools like labyrinth6, vortex7 and spiral8 chips have shown great outcomes in isolating CTCs. Since the number of cancer cells is very low in blood we need to choose a technique that can process blood at a high throughput manner with high yield and purity. Labyrinth device seems to be the right choice as it can process 2.5 mL blood per minutes, has a high yield (>90%) and purity (600 WBCs/mL). After separation of cancer cells from the blood next step would be identifying the cancer cells from the background white blood cells (WBCs). Current technique to identify cancer cell is immunostaining which is invasive and kills the cells barring further downstream analysis. To overcome this barrier researchers are trying to develop label-free techniques to identify cancer cells. For example, I am now working with Prof. Siva Vanapalli at Texas Tech University to develop deformability and optical property based techniques using digital holographic microscopy(DHM) to identify cancer cells. Immunostaining aliquot can be another way to handle the problem.
To understand the molecular basis of cancer and to select a specific therapy for a patient we need to combine the phenotypic data (whole cell) with genetic information. So, the next component of the workflow will include tools capable of phenotyping and genotyping at single cell resolution. Deformability cytometers (DCs)9-11 developed in recent years have the capability to phenotype single cells based on deformability at very high throughput (100 cells/sec) and identify the subpopulations to address heterogeneity. Real-time deformability cytometer (RT-DC)9 can generate deformability data for single cells in real-time while multi-sample deformability cytometer (MS-DC)11 can analyze 10 patient samples in a single experiment. The beauty of these deformability cytometers is that they are noninvasive and label-free. Cells collected from the outlet of DC devices can be used for genotyping. Microfluidic tools are also capable of genotyping cells as successfully demonstrated by Sohni et al.12 and Chang et al.13
Another component of the microfluidic tool for personalized cancer therapy should have is drug response analysis to avoid unnecessary or ineffective treatment. A study shows that medical waste due to unnecessary or ineffective treatment in the US per year is 75 billion US dollars14. The microfluidic capability of doing drug response analysis is well established. Bithi et al.15 isolated single cancer cells into microfluidic chambers and tested the drug response and uptake kinetics. Park et al16. studied the cellular response to the small-molecule drug within a microfluidic dielectrophoresis device in real-time.
The challenges for combining the individual tools together will be multifold as the operating conditions, sample preparation, addition of reagents and data analysis for each of them are different. Individual devices designed for a specific application may require certain modifications to be compatible with the workflow of personalized cancer therapy tool and real-world applications. Full process will require automation through artificial intelligence (AI), machine learning (ML) and image processing. I believe the day is not too far when we will see microfluidics is delivering as promised.
1. MD Anderson Cancer Center. Personalized cancer therapy (2018).
2. Terry, S. C., Jerman, J. H. & Angell, J. B. A gas chromatographic air analyzer fabricated on a silicon wafer. IEEE Transactions on Electron Devices 26, 1880-1886, doi:10.1109/T-ED.1979.19791 (1979).
3. Zhang, Z. & Nagrath, S. Microfluidics and cancer: are we there yet? Biomedical microdevices 15, 595-609, doi:10.1007/s10544-012-9734-8 (2013).
4. Hosic, S., Murthy, S. K. & Koppes, A. N. Microfluidic Sample Preparation for Single Cell Analysis. Analytical chemistry 88, 354-380, doi:10.1021/acs.analchem.5b04077 (2016).
5. Hattersley, S. M., Dyer, C. E., Greenman, J. & Haswell, S. J. Development of a microfluidic device for the maintenance and interrogation of viable tissue biopsies. Lab Chip 8, 1842-1846, doi:10.1039/b809345h (2008).
6. Lin, E. et al. High-Throughput Microfluidic Labyrinth for the Label-free Isolation of Circulating Tumor Cells. Cell Systems 5, 295-304.e294, doi:10.1016/j.cels.2017.08.012 (2017).
7. Che, J., Yu, V., Garon, E. B., Goldman, J. W. & Di Carlo, D. Biophysical isolation and identification of circulating tumor cells. Lab on a Chip 17, 1452-1461, doi:10.1039/C7LC00038C (2017).
8. Di Carlo, D. Inertial microfluidics. Lab on a Chip 9, 3038-3046, doi:10.1039/B912547G (2009).
9. Otto, O. et al. Real-time deformability cytometry: on-the-fly cell mechanical phenotyping. Nat Meth 12, 199-202, doi:10.1038/nmeth.3281 (2015).
10. Gossett, D. R. et al. Hydrodynamic stretching of single cells for large population mechanical phenotyping. Proceedings of the National Academy of Sciences 109, 7630-7635, doi:10.1073/pnas.1200107109 (2012).
11. Ahmmed, S. M. et al. Multi-sample deformability cytometry of cancer cells. APL Bioengineering 2, 032002, doi:10.1063/1.5020992 (2018).
12. Sohni, Y. R., Burke, J. P., Dyck, P. J. & O’Kane, D. J. Microfluidic chip-based method for genotyping microsatellites, VNTRs and insertion/deletion polymorphisms. Clinical Biochemistry 36, 35-40, doi:https://doi.org/10.1016/S0009-9120(02)00420-4 (2003).
13. Chang, Y.-M., Ding, S.-T., Lin, E.-C., Wang, L. & Lu, Y.-W. A microfluidic chip for rapid single nucleotide polymorphism (SNP) genotyping using primer extension on microbeads. Sensors and Actuators B: Chemical 246, 215-224, doi:https://doi.org/10.1016/j.snb.2017.01.160 (2017).
14. Lowrey, A. Study of US Health Care System Finds Both Waste and Opportunity to Improve (2012, Sep 11).
15. Bithi, S. S. & Vanapalli, S. A. Microfluidic cell isolation technology for drug testing of single tumor cells and their clusters. Scientific Reports 7, 41707, doi:10.1038/srep41707 (2017).
16. Park, I. S. et al. Real-Time Analysis of Cellular Response to Small-Molecule Drugs within a Microfluidic Dielectrophoresis Device. Anal Chem 87, 5914-5920, doi:10.1021/ac5041549 (2015).
Enjoyed this article? Don’t forget to share.
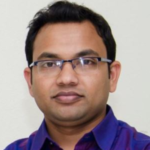
Shamim Ahmmed
Shamim Ahmmed is a final year Ph.D. student at microfluidics lab lead by Prof. Siva Vanapalli at Texas Tech University. His training is in Chemical Engineering, microfluidics and image processing. Shamim has unique skills for developing microfluidic tools for phenotyping cancer cells. He led three microfluidic projects from inception to publishing results by collaborating with three cross-functional groups which resulted in multiple publications and presentations. Shamim’s current project involves developing a microfluidic tool that can enumerate circulating tumor cells based on deformability and confirm that through on-chip immunostaining.